RKNN (Rockchip Neural Network) is a deep learning inference framework for embedded devices. It provides an end-to-end solution for transforming trained deep-learning models into executable files that run on embedded devices. The RKNN framework can efficiently run deep learning models on embedded devices, which is useful for application scenarios that require real-time inference on resource-constrained devices.
For example, RKNN can be used in smart cameras, robots, drones and other embedded devices to achieve artificial intelligence functions such as object detection, face recognition, and image classification, etc.
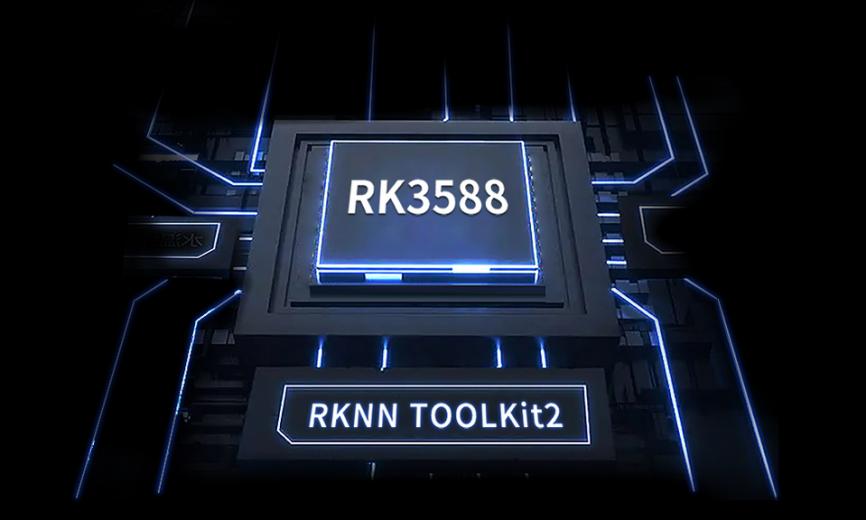
Let’s take an example to make the introduction: we use the RKNN-Toolkit2 tool to transform the yolov5s.onnx model in the rknpu2 project to a yolov5s.rknn model.
Connect it to the TypeC0 port of the board by a USB-typeC cable. Then it’s recognized in the virtual machine by the PC.
Check connection status in the development environment.
If the connection is successful, the device ID will be sent back, as follows:
Run rknn_server service on OK3588-C development board.
Check the running status of rknn_server in the development environment.
A return PID indicates successful running.
Modify test.py
Add target_platform='rk3588' to rknn.config
Add target='rk3588' to rknn.init_runtime
Run test.py after modification.
A successful run is as follows:
As well the yolov5s.rknn model is generated in the directory.
Execute the compile script to compile:
Then rknn_yolov5_demo is generated in the directory: rknpu2/examples/rknn_yolov5_demo/install/rknn_yolov5_demo_Linux
Go to the directory: rknn_yolov5_demo_Linux and add the environment variables for the linked libraries (lib directory in the rknn_yolov5_demo_Linux directory)
Use rknn model to conduct object recognition commands as follows:
Execution results:
Copy the generated out.jpg to the local computer to review the recognition results:
That’s all the procedure of transforming and testing the RK3588 inference model based on Forlinx OK3588-C development board.
Originally published at www.forlinx.net.
For example, RKNN can be used in smart cameras, robots, drones and other embedded devices to achieve artificial intelligence functions such as object detection, face recognition, and image classification, etc.
Let’s take an example to make the introduction: we use the RKNN-Toolkit2 tool to transform the yolov5s.onnx model in the rknpu2 project to a yolov5s.rknn model.
01 Download RKNN-Toolkit2
02 Installation Lib
requirements_cp36-1.3.0.txt is in the rknn-toolkit2/doc directory:03 Connect Development Environment to OK3588-C Development Board.
Install adb in the development environment.Connect it to the TypeC0 port of the board by a USB-typeC cable. Then it’s recognized in the virtual machine by the PC.
Check connection status in the development environment.
If the connection is successful, the device ID will be sent back, as follows:
04 Download NPU Project
05 Send rknn_server and rknn library to the Development Board.
Run rknn_server service on OK3588-C development board.
Check the running status of rknn_server in the development environment.
A return PID indicates successful running.
06 Model Transformation
Enter the development environment→rknn_toolkit2→examples directory→select a model. In this example, we choose to transform the onnx model to an RKNN model.Modify test.py
Add target_platform='rk3588' to rknn.config
Add target='rk3588' to rknn.init_runtime
Run test.py after modification.
A successful run is as follows:
As well the yolov5s.rknn model is generated in the directory.
07 Compile and Test the Source Code
Go to the dirctory: rknpu2/examples/rknn_yolov5_demo and set the environment variables:Execute the compile script to compile:
Then rknn_yolov5_demo is generated in the directory: rknpu2/examples/rknn_yolov5_demo/install/rknn_yolov5_demo_Linux
08 Test
Copy the yolov5s.rknn model generated above and the rknn_yolov5_demo_Linux in the install directory to the development board.Go to the directory: rknn_yolov5_demo_Linux and add the environment variables for the linked libraries (lib directory in the rknn_yolov5_demo_Linux directory)
Use rknn model to conduct object recognition commands as follows:
Execution results:
Copy the generated out.jpg to the local computer to review the recognition results:
That’s all the procedure of transforming and testing the RK3588 inference model based on Forlinx OK3588-C development board.
Originally published at www.forlinx.net.